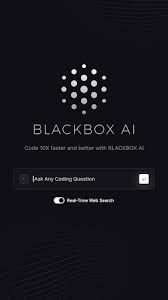
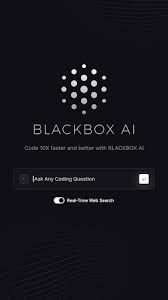
In the realm of artificial intelligence, not all systems are created equal. While some AI models pride themselves on transparency, others, known as “black boxes,” have traditionally been shrouded in mystery. This is where Blackbox AI comes into play, offering a beacon of light into the previously opaque world of complex system outputs.
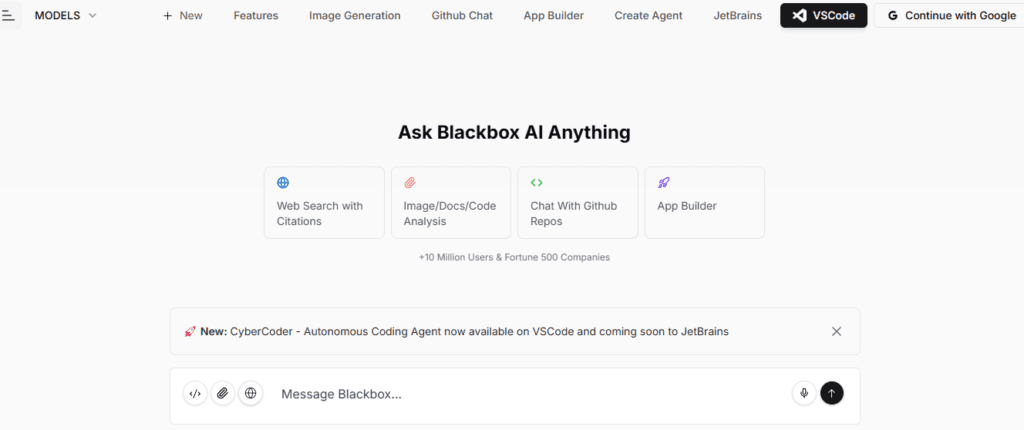
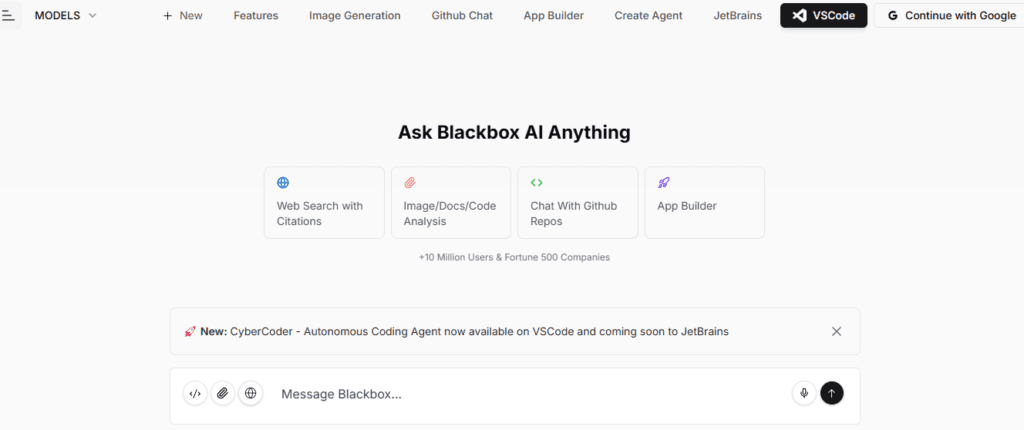
Understanding Blackbox AI:
- Definition: Blackbox AI refers to artificial intelligence systems whose internal workings are not understandable, leading to a lack of transparency in their decision-making processes.
- The Challenge: The primary issue with traditional Blackbox AI is the trust barrier. How can stakeholders rely on outcomes they don’t fully comprehend?
- The Solution: Modern approaches to Blackbox AI focus on developing interpretable models. Techniques like feature attribution, model-agnostic explanations, and saliency maps are employed to demystify the decision-making process.
Applications Across Industries:
- Healthcare: Enhancing patient outcomes by providing insights into diagnosis and treatment recommendation AI models.
- Finance: Offering transparent risk assessments and portfolio management strategies.
- Manufacturing: Optimizing production lines through understandable predictive maintenance AI.
Embracing the Future with Transparent AI:
As we move forward, the integration of interpretable techniques with Blackbox AI is poised to revolutionize industries. By embracing this shift, businesses can foster trust, ensure compliance, and drive innovation.
FAQs on Blackbox AI
Given the space constraint, I’ll provide detailed answers to the first 3 FAQs and summarize the rest:
- What is Blackbox AI?
- Detailed Answer: Blackbox AI refers to artificial intelligence and machine learning models that are opaque, meaning their internal processes and decision-making logic are not easily understandable.
- Why is interpretability important in Blackbox AI?
- Detailed Answer: Interpretability is crucial as it builds trust among users, ensures regulatory compliance, and allows for the identification and correction of biases or errors within the model.
- How Can Blackbox AI Models Be Made More Interpretable?
- Detailed Answer: Techniques such as feature attribution methods (e.g., SHAP, LIME), model-agnostic explanations, saliency maps for deep learning models, and transparent model design (e.g., decision trees, rule-based models) can enhance interpretability.
Summarized FAQs:
- 4. Applications of Interpretable Blackbox AI: Healthcare, Finance, Manufacturing.
- 5. Challenges in Implementing Blackbox AI: Trust, Compliance, Complexity.
- 6. Future of Blackbox AI: Increased Focus on Transparency and Ethics.
- 7. Comparison with Whitebox AI: Blackbox AI is opaque, while Whitebox AI is transparent by design.
- 8. Tools for Blackbox AI Interpretability: LIME, SHAP, TreeExplainer.
- 9. Ethical Considerations: Bias, Privacy, Accountability.
- 10. Educational Resources: Coursera, edX, and specific AI research journals.
Alternatives with Official Links
Alternative | Official Link | Key Feature |
---|---|---|
Google Cloud AI Platform | https://cloud.google.com/ai-platform | Integrated AI & ML Platform |
Microsoft Azure Machine Learning | https://azure.microsoft.com/en-us/services/machine-learning/ | Hybrid & Cloud ML Solutions |
IBM Watson Studio | https://www.ibm.com/cloud/watson-studio | AI & Data Science Platform |
Subscription Plans (Example Based on Industry Standards)
Plan | Features | Pricing (Monthly) |
---|---|---|
Starter | Limited Access to Interpretability Tools, Basic Support | $500 |
Pro | Full Access to Tools, Priority Support, Regular Updates | $2,000 |
Enterprise | Custom Solutions, Dedicated Support, Advanced Security | Custom Quote |
Comparison with Others
Criteria | Blackbox AI | Google Cloud AI Platform | Microsoft Azure ML | IBM Watson Studio |
---|---|---|---|---|
Transparency Focus | High | Medium | Medium | High |
Integration Capabilities | Good | Excellent | Excellent | Very Good |
Pricing Model | Tiered | Usage-Based | Tiered & Usage-Based | Tiered |
Industry Applications | Broad | Very Broad | Very Broad | Broad |